AI Unlocked: Siphoning and Scams in the Age of Artificial Intelligence
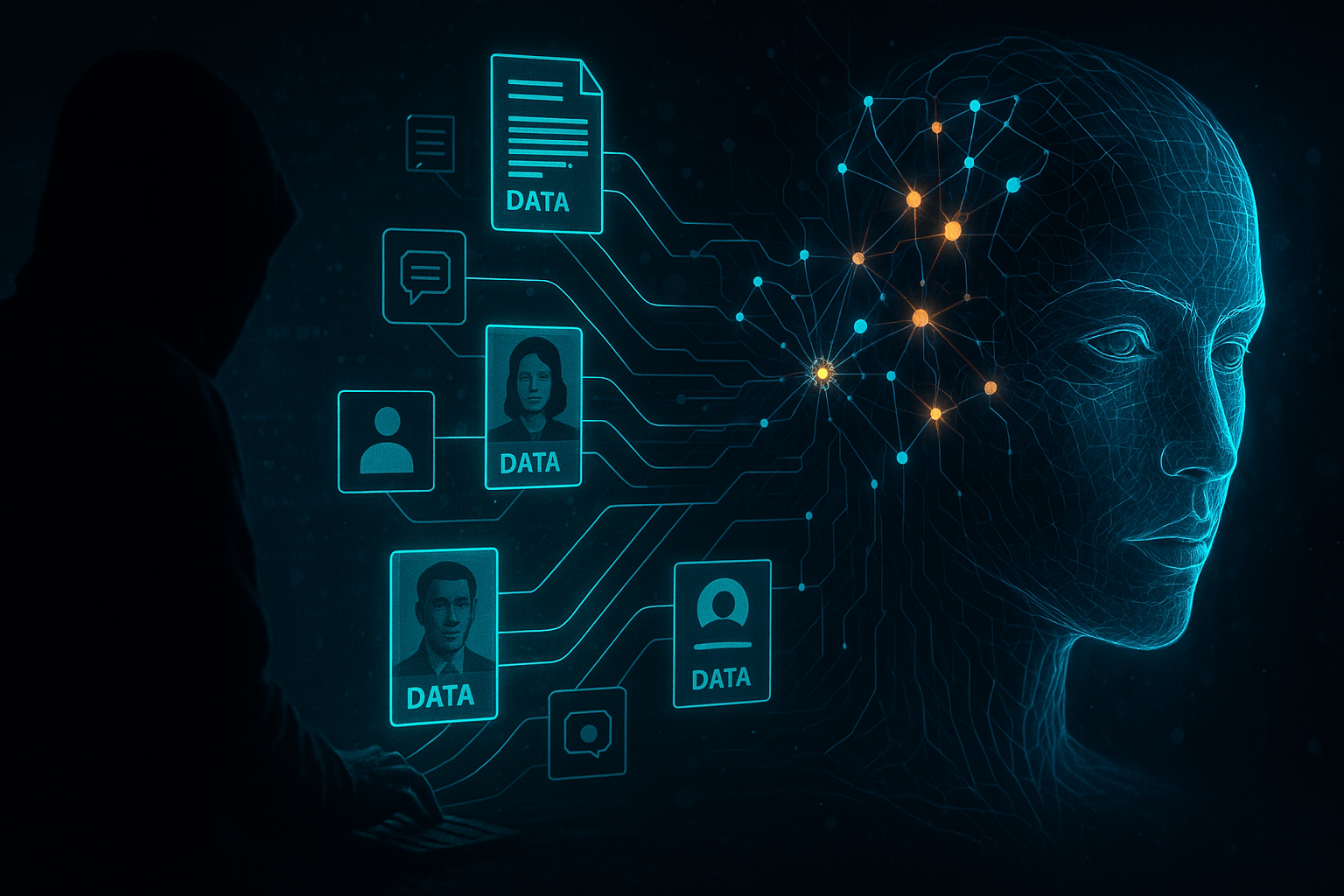
The Hidden Data Economy: How Your Information Powers AI and Empowers Fraudsters
In today's rapidly evolving digital landscape, artificial intelligence has transformed from a futuristic concept to an everyday reality. Behind this technological revolution lies an uncomfortable truth: the massive collection of personal and business data, often without explicit permission, that fuels these systems. This practice, sometimes called "Deep Seek" siphoning, represents both a technological breakthrough and a significant risk to individuals and businesses across North America.
For Canadian and American business leaders, understanding the mechanics of AI data siphoning isn't just academic—it's essential for corporate security, compliance, and risk management. For consumers, knowledge about how their data is being harvested may be the first line of defense against increasingly sophisticated AI-powered scams.
The Mechanics of AI Data Siphoning
Data siphoning for AI isn't merely collecting information—it's about mass harvesting content from across the internet to train sophisticated machine learning models. This collection happens through several primary channels:
Web Scraping at Scale
AI development companies employ advanced web crawlers that systematically visit websites, forums, social platforms, and online repositories to collect text, images, videos, and code. Unlike traditional crawlers that index content for search engines, these specialized bots extract and store the actual content for AI training purposes.
"What many business leaders fail to understand is that their company websites, including product descriptions, customer testimonials, and technical documentation, are being harvested daily to train competitor AI systems," explains Dr. Eliana Chen, cybersecurity analyst at Northern Shield Technologies in Toronto. "This happens without notification or compensation."
API Access and Data Partnerships
Beyond direct scraping, some AI developers gain access to data through APIs or formal partnerships with data brokers, social media platforms, and other digital services. These arrangements sometimes operate in regulatory gray areas, particularly when the original users were unaware their information would be shared for AI training.
User-Generated Content
Perhaps most concerning is the harvesting of user-generated content: social media posts, product reviews, uploaded photos, videos, and comments. This content, rich in personal perspectives, writing styles, preferences, and even biometric data like faces and voices, forms the foundation of many AI training datasets.
The Business Impact: When Your Content Becomes Training Data
For businesses, the implications of widespread data siphoning extend far beyond privacy concerns:
Intellectual Property Concerns
Companies invest significant resources in creating original content for websites, marketing materials, and technical documentation. When this material is siphoned without permission to train AI systems, it effectively transforms proprietary content into training data that may benefit competitors or become embedded in publicly available AI tools.
"We discovered sections of our proprietary product documentation appearing verbatim in AI-generated content," says Marcus Williams, CTO of Vancouver-based software firm Quantum Solutions. "Essentially, our competitors were receiving the benefit of our R&D and documentation investments through AI tools trained on our content."
Compliance Vulnerabilities
As regulations like Canada's PIPEDA, Quebec's Bill 64, and various U.S. state data protection laws continue to evolve, businesses face potential liability if customer data they've collected is subsequently siphoned and used for AI training. This raises complex questions about adequate security measures and proper disclosure.
Competitive Disadvantage
When industry-specific data is harvested en masse, businesses that invested heavily in developing proprietary datasets may find their competitive advantage diminished as similar insights become available through AI tools trained on their information.
AI-Powered Scams: The Dark Side of Data Siphoning
The same data that powers legitimate AI applications also enables increasingly sophisticated scams targeting both businesses and consumers:
Voice Cloning and Deepfake Fraud
Perhaps the most alarming development is voice cloning technology. With just a few minutes of recorded speech—easily gathered from conference presentations, earnings calls, podcasts, or social media videos—fraudsters can create convincing voice clones capable of deceiving colleagues, employees, or family members.
"Last quarter, we documented 37 cases of executive voice clone fraud attempts against Fortune 1000 companies," notes Raymond Ortiz, fraud prevention specialist at Deloitte. "In three cases, the attacks resulted in successful wire transfers averaging $285,000 before being detected."
These attacks typically follow a similar pattern: 1. A seemingly urgent call from the CEO or CFO to a finance team member 2. A request for an immediate, confidential wire transfer 3. A plausible explanation for why normal protocols can't be followed 4. Social pressure and urgency that discourage verification
Ultra-Personalized Phishing
Traditional phishing attacks cast a wide net with generic messages. AI-powered phishing uses siphoned data to craft highly personalized messages that reference specific projects, colleagues, or recent events within an organization—dramatically increasing success rates.
"The personalization level we're seeing is unprecedented," explains Jennifer Kim, Director of Information Security at American Express. "These aren't just emails addressing you by name—they reference specific meetings you attended last week, use the exact linguistic patterns of your colleagues, and arrive contextually timed around related business events."
Data-Enriched Social Engineering
Social engineering—the psychological manipulation techniques used to trick people into breaking security protocols—becomes exponentially more effective when powered by AI analysis of siphoned data. Fraudsters can identify organizational hierarchies, personal relationships, communication patterns, and even individual psychological vulnerabilities.
The Consumer Perspective: Your Digital Shadow
For everyday consumers, the reality of data siphoning manifests in several concerning ways:
Identity Projection and Theft
As AI systems ingest social media histories, public records, and online activities, they can effectively project potential future behaviors, preferences, and even location patterns. This level of prediction makes identity theft and targeted scams significantly more convincing.
"What's particularly troubling is how AI can fill in the gaps in someone's digital profile," says privacy advocate Thomas Morrison. "Even if you've only shared discrete pieces of information across different platforms, AI systems can correlate and complete that picture in ways traditional data analysis never could."
Perpetual Data Retention
Unlike traditional data collection, which might be subject to retention limitations, siphoned data used for AI training often becomes permanently embedded in the weights and parameters of machine learning models. This creates a situation where personal information essentially cannot be deleted once it's been incorporated into AI systems.
Cross-Platform Correlation
Data siphoned from multiple sources allows AI systems to correlate information across platforms, creating comprehensive profiles that exceed what any single service could collect. This enables identification and targeting even when using supposedly separate or anonymous accounts.
Legal and Regulatory Landscape
The regulatory environment surrounding AI data siphoning remains fragmented and evolving:
Canadian Context
In Canada, the Personal Information Protection and Electronic Documents Act (PIPEDA) requires informed consent for the collection, use, and disclosure of personal information. However, its application to AI training data remains ambiguous, particularly when information is gathered from public sources.
Quebec's Bill 64, which came into full effect in September 2023, provides more explicit AI governance, requiring impact assessments for automated decision systems and enhanced transparency around how personal information is used.
U.S. Landscape
The United States lacks comprehensive federal privacy legislation specifically addressing AI data collection. Instead, a patchwork of state laws creates varying protections:
- California's Consumer Privacy Act (CCPA) and Consumer Privacy Rights Act (CPRA) provide residents with rights to know what personal information is collected and how it's used, including for AI training.
- Illinois' Biometric Information Privacy Act (BIPA) requires explicit consent before collecting biometric identifiers, leading to successful litigation against companies that used facial recognition in AI training without permission.
- Several states are considering or have passed more targeted AI regulations, particularly around disclosure of AI-generated content.
Corporate Protection Strategies
For businesses concerned about data siphoning, several protective measures can reduce risk:
Technical Countermeasures
- Implement robots.txt directives specifically addressing AI crawlers, though be aware compliance is voluntary
- Deploy CAPTCHA and rate-limiting on publicly accessible content to reduce automated scraping
- Use digital watermarking for proprietary images and content
- Consider dynamic content loading that makes automated collection more difficult
Legal Protections
- Review and update Terms of Service to explicitly prohibit automated data collection and use of content for AI training
- Implement clear data access agreements with vendors and partners that specify limitations on AI training use
- Consider registration of key content with copyright authorities
- Document your original content creation process to establish clear provenance
Operational Security
- Conduct regular AI impersonation testing to identify vulnerabilities
- Implement multi-factor verification protocols for financial transactions
- Train employees on voice clone and deepfake detection
- Establish out-of-band verification channels for high-risk requests
Consumer Protection Strategies
Individuals concerned about their data being siphoned can take several protective steps:
Active Digital Hygiene
- Regularly audit privacy settings across platforms
- Limit public-facing personal information
- Consider using pseudonyms for non-essential services
- Be selective about biometric data sharing (voice recordings, facial recognition)
Verification Protocols
- Establish verification codes or questions with family members for urgent requests
- Use callback verification for unexpected financial requests
- Be skeptical of urgency in financial or personal information requests
- Verify through alternative channels when receiving unusual requests from known contacts
Awareness and Education
- Stay informed about current AI impersonation techniques
- Learn to recognize signs of synthetic media
- Understand that public content may be permanent once collected
- Advocate for stronger privacy protections and corporate responsibility
The Future of Data Siphoning and AI Security
As we look ahead, several trends are likely to shape the landscape of AI data collection and associated risks:
Regulatory Evolution
Expect significant development in both Canadian and U.S. regulatory frameworks specifically addressing AI training data collection, with potential requirements for: - Explicit consent for using content in AI training - Transparency in identifying AI-generated content - Mandatory disclosure of data sources used in AI development - Right to removal from training datasets
Technological Arms Race
The competition between data collection technologies and protective measures will accelerate: - More sophisticated scraping technologies that can bypass traditional protections - Advanced AI detection tools that can identify synthetic content - Blockchain-based content verification systems - Federated learning approaches that reduce need for centralized data collection
Market-Based Solutions
Market forces may drive the development of: - Opt-in platforms that compensate content creators for AI training use - Premium AI services that use only properly licensed training data - Data provenance services that track content origins - Insurance products specifically covering AI impersonation risks
Conclusion: Vigilance in the Age of AI
The rapid advancement of artificial intelligence creates both remarkable opportunities and significant risks for businesses and consumers across North America. Data siphoning—the massive, often unconsented collection of digital content—powers this revolution but also enables sophisticated fraud and raises profound questions about ownership, consent, and digital rights.
For business leaders, protecting corporate digital assets while leveraging AI capabilities requires a balanced approach combining technical safeguards, legal protections, and operational awareness. For consumers, understanding the value and vulnerability of personal data is essential for navigating an increasingly AI-mediated world.
As regulations evolve and technical countermeasures improve, one thing remains clear: awareness and education represent the first and most effective line of defense against the dark side of AI advancement. By understanding how data siphoning works and implementing appropriate protections, both businesses and individuals can enjoy the benefits of AI while mitigating its most significant risks.
About AI Unlocked: This article is part of our ongoing series examining the practical implications of artificial intelligence for North American businesses and consumers.